Understanding Data Labeling: The Key to Effective Machine Learning
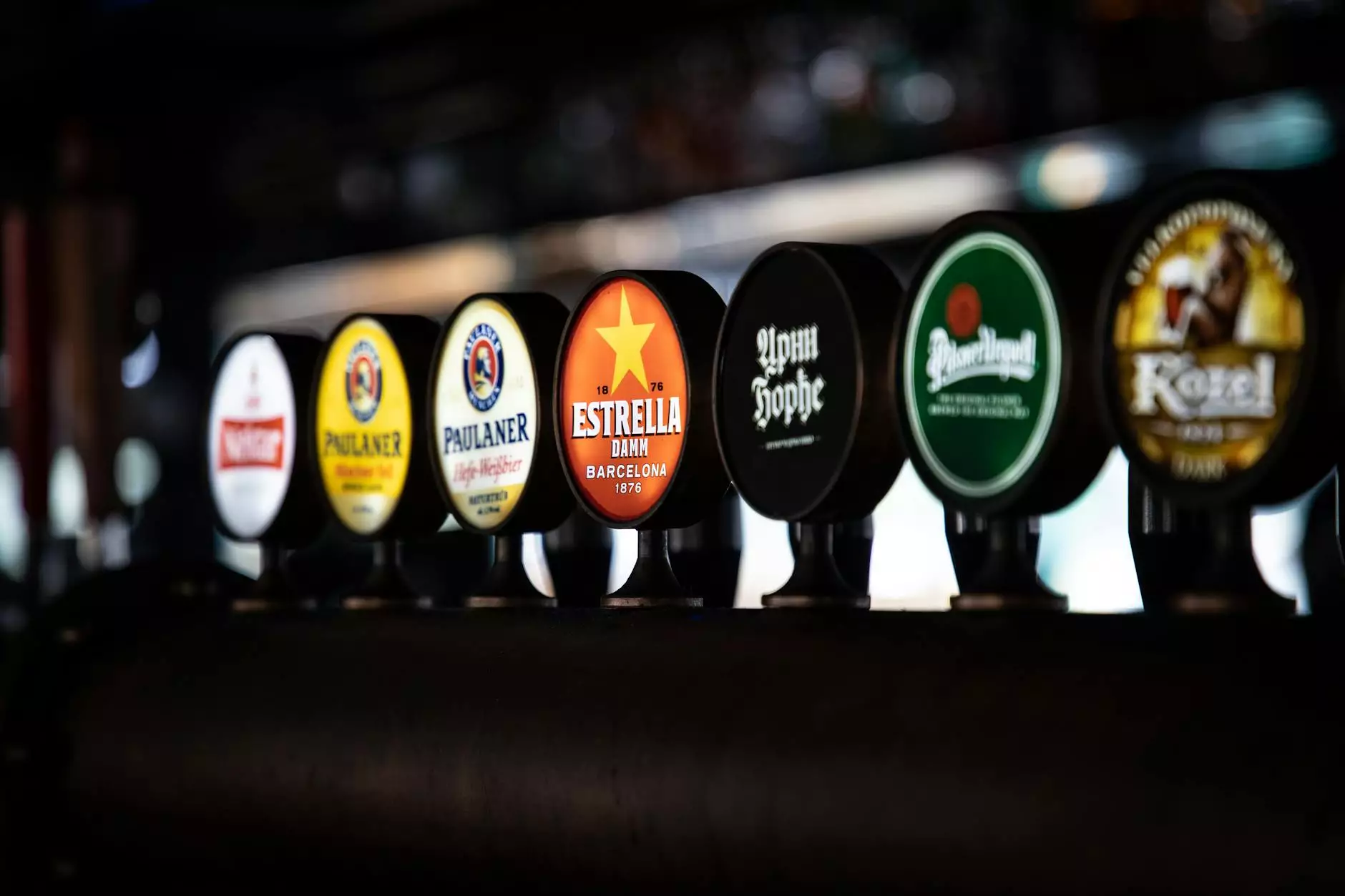
In the digital age, businesses are increasingly reliant on the power of artificial intelligence (AI) and machine learning (ML) to drive decision-making, enhance customer experience, and gain a competitive edge. One of the fundamental aspects that underpin the success of machine learning is data labeling. In this article, we will delve deep into what data labeling is, its significance, the methodologies involved, and how platforms like keylabs.ai can facilitate effective data annotation.
What is Data Labeling?
Data labeling is the process of categorizing and tagging data to inform models in machine learning and AI applications. Essentially, it involves providing meaningful context to data, which could be anything from text, images, and videos to audio files. This process allows algorithms to learn from the data and make predictions or decisions based on the annotated information.
Why is Data Labeling Important?
The importance of data labeling cannot be overstated. Here are several reasons why it is crucial in the realms of AI and ML:
- Training AI Models: Data labeling is essential for creating high-quality training datasets. Well-labeled data helps algorithms discern patterns and learn effectively.
- Improving Accuracy: High-quality, accurately labeled data leads to better model performance, yielding more reliable predictions and insights.
- Domain-Specific Applications: Different industries require specific data interpretations. For example, in healthcare, correctly labeled imaging data can significantly enhance diagnostic accuracy.
- Automating System Processes: Organizations can utilize labeled data to automate complex processes, improving overall efficiency.
The Data Labeling Process
Understanding the data labeling process is imperative for businesses looking to implement effective machine learning strategies. The process can be segregated into several key stages:
1. Data Collection
The first step involves gathering diverse datasets that are representative of the problem domain. This data can come from numerous sources including databases, public datasets, or user-generated content.
2. Data Annotation
Data annotation encompasses various techniques to label data accurately. Different data types require distinct labeling methods, which include:
- Image Labeling: Tagging images with objects, attributes, or classifications (e.g., bounding boxes or pixel-wise segmentation).
- Text Annotation: Involves labeling text data with semantic meanings (e.g., identifying sentiment, keyword extraction).
- Audio Annotation: Tagging audio clips with transcriptions or sound classification (e.g., speech recognition or noise detection).
- Video Annotation: Similar to image labeling but considers temporal aspects (e.g., frame-by-frame tagging for object tracking).
3. Quality Assurance
One of the critical aspects of data labeling is ensuring quality. This stage may involve multiple rounds of review, leveraging automated tools or human reviewers to validate label accuracy.
4. Model Training
Once the data is labeled and verified, it can be fed into machine learning models for training, enabling them to learn and improve their predictive capabilities.
Challenges in Data Labeling
While data labeling is crucial, it is not without challenges. Here are common hurdles that organizations face:
- Scalability: The demand for labeled data can outpace available resources, making it difficult to manage large datasets efficiently.
- Data Privacy: Handling sensitive data poses risks and regulatory challenges, necessitating robust data protection protocols.
- Consistency: Ensuring consistent labeling across various team members can be a daunting task, impacting the data’s reliability.
- Cost: High-quality data labeling can be expensive, particularly when outsourcing to specialized firms.
Data Labeling Tools and Platforms
Given the intricate nature of data labeling, utilizing specialized tools and platforms can vastly improve efficiencies. keylabs.ai stands out as a premier solution in this space, providing comprehensive data annotation tools and platforms tailored for businesses seeking robust AI and ML applications.
Advantages of Using Data Annotation Tools
Here are reasons why employing a data annotation tool is beneficial:
- Streamlined Workflows: Tools often come with intuitive interfaces, allowing users to navigate data efficiently.
- Automation: Many platforms utilize AI-assisted data labeling, reducing the manual effort required and expediting the process.
- Collaboration Features: Data annotation platforms facilitate teamwork by allowing multiple users to work on datasets simultaneously.
- Customizability: Businesses can often customize annotation settings and workflows to match their specific requirements.
The Future of Data Labeling
The field of data labeling is incredibly dynamic and continues to evolve as machine learning advancements are made. Here are some trends to keep an eye on:
1. Increased Automation
As machine learning algorithms become more sophisticated, automating data labeling processes will become standard practice. This could reduce costs and time significantly while enhancing accuracy.
2. Crowdsourcing Annotation Tasks
Crowdsourcing could prove essential in tapping into a diverse pool of labelers, thus accelerating the labeling process. This method emphasizes leveraging the "wisdom of the crowd" for varied insights and interpretations.
3. Enhanced Quality Control Mechanisms
The future of data labeling will likely see the integration of advanced validation tools to ensure quality control, utilizing algorithms to detect inconsistencies and inaccuracies.
4. Domain-Specific Tools
As the demand for tailored AI solutions rises, we can anticipate the emergence of specialized data labeling tools designed for specific industries, such as healthcare, finance, and autonomous driving.
Conclusion
In the world of machine learning, data labeling is not just a preliminary step; it's the foundation upon which AI applications are built. By investing in high-quality data annotation, businesses like keylabs.ai can propel their machine learning initiatives forward, ensuring accurate, reliable, and actionable insights. As this field continues to evolve, staying abreast of trends and utilizing advanced tools will be crucial for success. With the right strategies and resources in place, organizations can unlock the full potential of their data and harness AI's capabilities to drive growth and innovation.