Revolutionizing Agriculture: The Role of Machine Learning Datasets
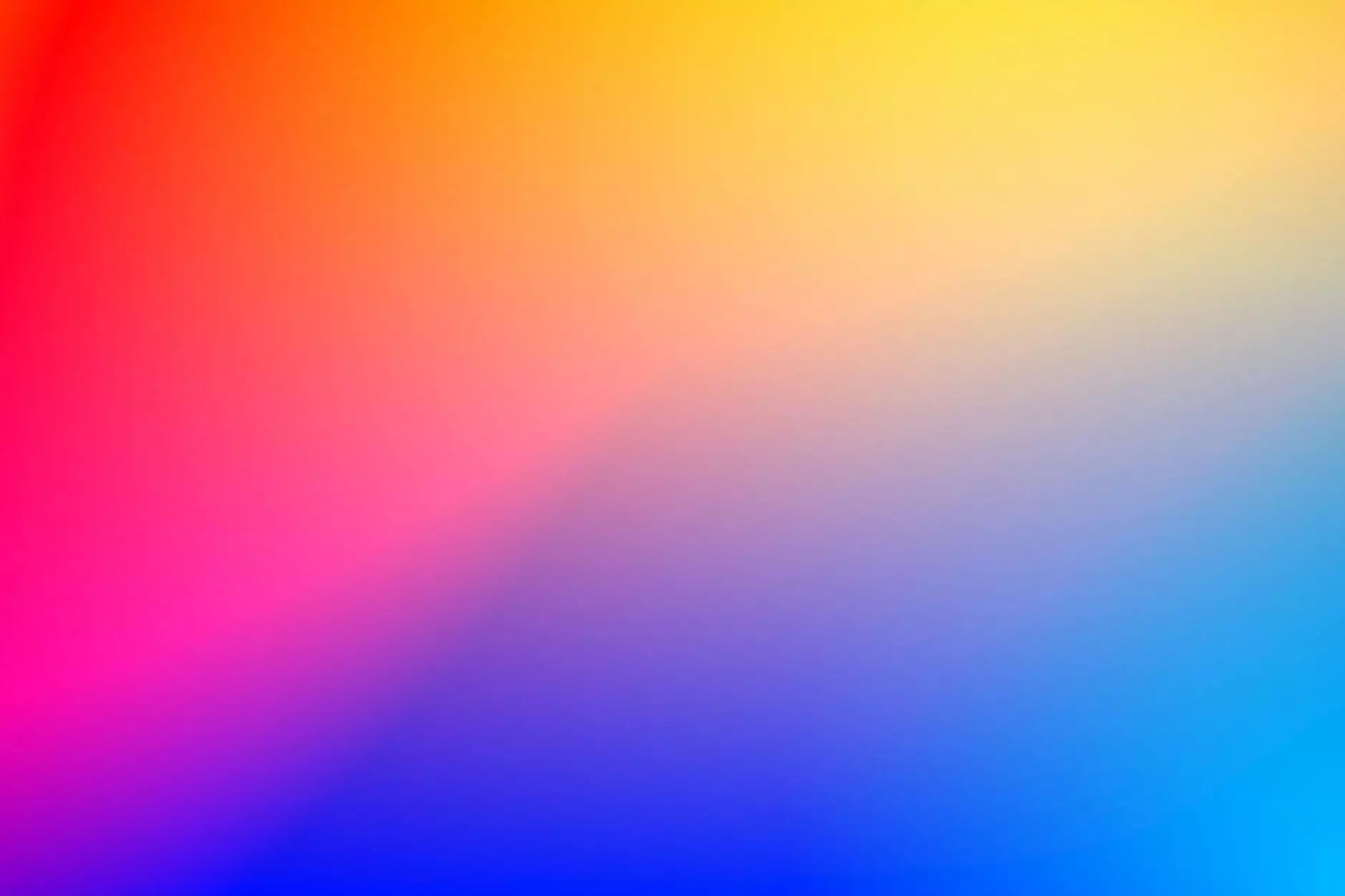
Agriculture is one of the oldest and most essential industries in human history. As we enter the age of digital transformation, the convergence of technology and agriculture is becoming increasingly vital. Among the most impactful innovations in this space is the use of machine learning, particularly through the utilization of comprehensive agriculture datasets for machine learning. In this article, we will explore the transformative potential of these datasets and how they can empower modern farming practices.
The Significance of Agriculture Datasets
Agriculture datasets serve as the foundation for machine learning algorithms that can drive insights and efficiencies in farming. These datasets include a vast array of information related to crop yields, soil quality, weather patterns, pest management, and much more. By analyzing this data, farmers can make informed decisions that enhance productivity and sustainability.
Why Data Matters in Agriculture
- Informed Decision-Making: Access to accurate data helps farmers make better decisions regarding planting, harvesting, and resource allocation.
- Increased Efficiency: By understanding variables that affect yields, farmers can optimize their operations to reduce waste and costs.
- Sustainability: Data-driven practices lead to a more sustainable approach to farming by minimizing the impact on the environment.
- Competitive Edge: Utilizing data analytics and machine learning gives farmers a technological advantage over competitors who do not adopt these methods.
Types of Agriculture Datasets
Understanding different types of agriculture datasets is crucial for leveraging their full potential. Here are several key categories of datasets that are valuable for machine learning applications:
1. Crop Yield Data
Crop yield data provides information on the amount of agricultural produce harvested per unit area. This data is critical for predicting future yields, understanding the impact of weather patterns, and optimizing planting schedules. By analyzing past yield records alongside environmental conditions, machine learning models can forecast future performance and identify areas for improvement.
2. Soil Quality Data
Soil quality datasets encompass various factors such as pH levels, nutrient content, and moisture levels. These datasets facilitate the development of tailored fertilization and irrigation strategies, leading to healthier crops and optimized resource use. Machine learning models can identify correlations between soil quality indicators and crop performance, providing insights that can guide farming practices.
3. Weather and Climate Data
Weather conditions play a pivotal role in farming success. Datasets that include temperature, precipitation, humidity, and other climatic variables allow farmers to predict how weather patterns will affect their crops. Machine learning can analyze historical weather data to identify trends and potential agricultural risks, enabling proactive measures to mitigate these challenges.
4. Market and Economic Data
Data related to market trends, pricing, and economic indicators helps farmers make informed decisions about what crops to grow and when to sell their produce. Machine learning models can analyze market datasets to forecast demand and pricing trends, optimizing the timing of sales to maximize profits.
5. Pest and Disease Data
Pest and disease datasets highlight outbreaks and conditions that lead to crop damage. Analyzing this data through machine learning enables farmers to develop early warning systems, improving their ability to react quickly to these threats. This proactive approach can significantly reduce losses and enhance crop resilience.
Implementing Machine Learning in Agriculture
The successful implementation of machine learning in agriculture depends on several factors, including the availability of high-quality data, the right algorithms, and collaboration among stakeholders. Here’s how it can be effectively executed:
1. Data Collection and Management
The first step is to collect relevant data from various sources. This data must be aggregated, cleaned, and formatted for analysis. Organizations involved in agriculture should invest in robust data management systems to ensure that datasets are easily accessible and reliable.
2. Choosing the Right Algorithms
Machine learning encompasses numerous algorithms, each suited for different types of data and analytical tasks. For agricultural applications, algorithms such as regression analysis, decision trees, and clustering can help derive actionable insights. Selecting the right algorithm is essential for achieving accurate predictions and results.
3. Collaboration Across the Industry
Collaboration among farmers, researchers, and technology providers is crucial for driving innovation. By sharing data and insights, the agricultural community can develop best practices and improve the overall effectiveness of machine learning applications.
The Future of Agriculture with Machine Learning
The integration of machine learning into agriculture is not merely a trend; it represents a shift towards a more data-driven, efficient, and sustainable approach. Here's what the future may hold:
1. Precision Agriculture
Machine learning will enable the next generation of precision agriculture, where decisions are made based on real-time data analysis. This will optimize the use of inputs, leading to increased yields and reduced environmental impact.
2. Automated Farming Solutions
As machine learning algorithms advance, we will see more automated solutions in farming. Robots equipped with machine learning capabilities can monitor crops, apply fertilizers, and even harvest produce autonomously, reducing the need for manual labor.
3. Enhanced Crop Varieties
Machine learning can assist in agricultural biotechnology, aiding in the development of crop varieties that are resistant to diseases and extreme weather conditions. This innovation can significantly boost food security and adaptability in the face of climate change.
4. Global Food Security
Utilizing agriculture datasets for machine learning can contribute to achieving global food security. By improving yield predictions and resource management, we can ensure a more stable food supply, which is vital as the global population continues to grow.
Conclusion
In conclusion, the integration of agriculture datasets for machine learning is set to revolutionize the agricultural sector. The potential benefits are immense: from increased efficiency and sustainability to improved crop yields and enhanced decision-making capabilities. As stakeholders in the agriculture industry continue to embrace data-driven technologies, we are likely to see a remarkable transformation in how food is produced and managed globally. The future of farming is here, powered by data and machine learning.
To learn more about how keymarket innovations can enhance your business in agricultural technology, visit keymakr.com for extensive resources and support in embracing this technological shift.
agriculture dataset for machine learning