Unlocking Efficiency with an Automatic Image Annotation Tool
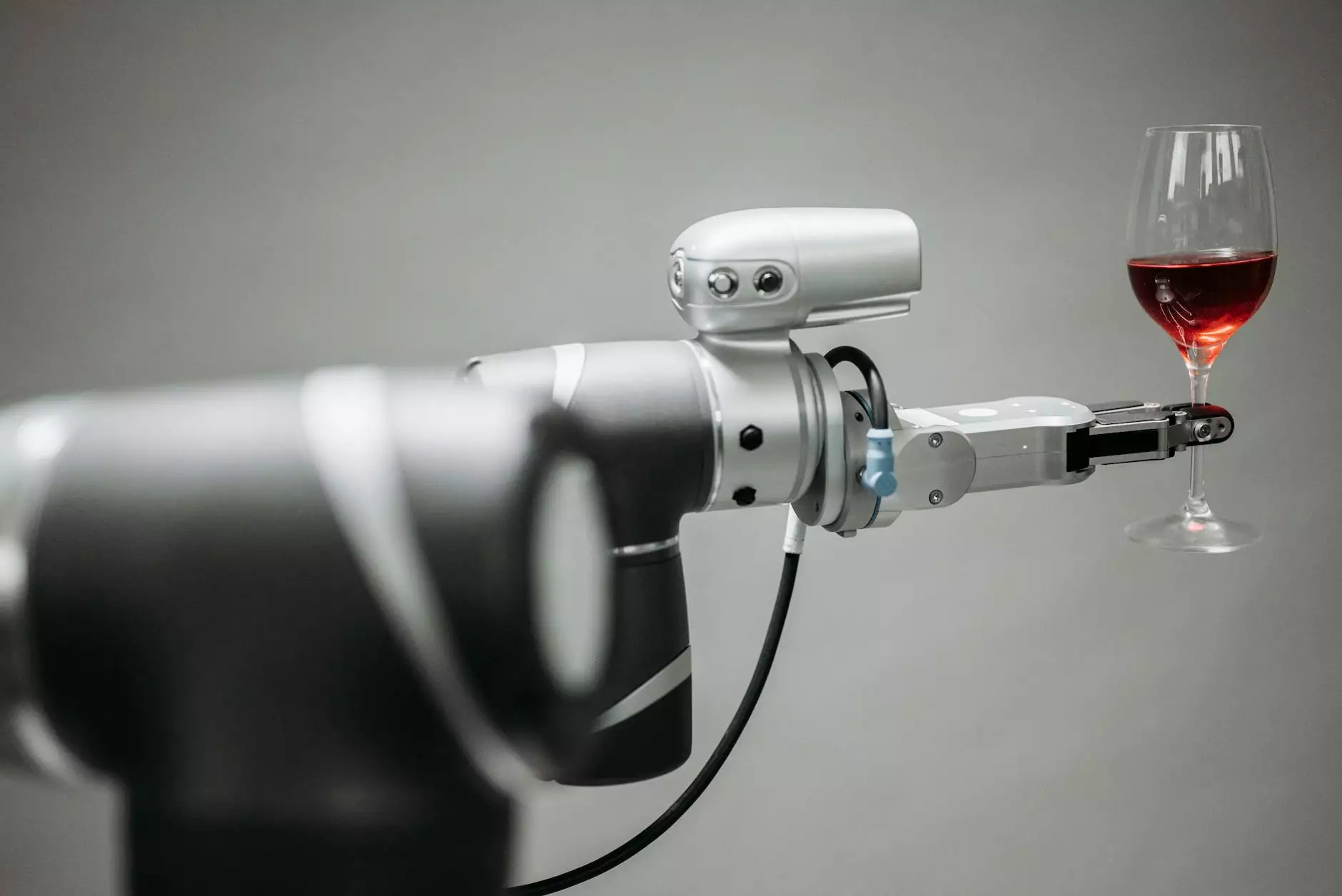
In today’s fast-paced digital landscape, businesses are increasingly relying on artificial intelligence (AI) and machine learning (ML) to streamline workflows and enhance productivity. One pivotal aspect of this technological evolution is the emergence of the automatic image annotation tool, which has revolutionized how we handle image data in various applications. With the ability to enhance software development processes, this tool offers a multitude of benefits that can significantly improve project outcomes.
What is an Automatic Image Annotation Tool?
An automatic image annotation tool is software designed to automatically label images based on predefined criteria or algorithms. This process helps in training machine learning models more effectively by providing them with rich, annotated datasets. By utilizing these tools, developers can save countless hours that would otherwise be spent manually annotating images.
Why is Image Annotation Important?
The importance of image annotation cannot be overstated in the realm of AI development. Here are several key reasons why it is critical:
- Data Preparation: Annotated images are essential for supervised learning models, allowing machines to learn from labeled data.
- Enhanced Accuracy: Properly annotated datasets lead to improved accuracy in predictions and classifications made by AI systems.
- Efficient Workflows: Automation reduces the need for manual intervention, freeing team members to focus on more strategic tasks.
- Scalability: An annotation tool enables projects to scale quickly without a linear increase in labor costs.
The Advantages of Using an Automatic Image Annotation Tool
Integrating an automatic image annotation tool into your software development process comes with a host of benefits. Let’s delve into some of the most significant advantages:
1. Time Savings
Time is one of the most precious resources in any business. The automatic image annotation tool drastically cuts down the time required for image labeling. By automating the process, companies can shift their focus from repetitive tasks to more critical aspects of development.
2. Cost Efficiency
Reducing the time spent on manual annotation translates directly into lower labor costs. With fewer resources allocated to data preparation, businesses can redirect those savings into other essential areas like research and development.
3. Increased Accuracy
While manual annotations can lead to human errors, automated tools typically employ sophisticated algorithms that enhance accuracy. This precision is especially crucial in applications like autonomous driving or medical imaging, where stakes are high, and errors can be costly.
4. Improved Collaboration
Using a centralized automatic image annotation tool fosters better collaboration among team members. With a cloud-based platform, all stakeholders can access annotated datasets, ensuring everyone is on the same page and promoting cohesive development efforts.
5. Robust Training Data
Machine learning models thrive on quality datasets. Automatic annotation tools produce a vast amount of labeled data, which is instrumental in training algorithms to perform accurately. The more diverse and comprehensive the dataset, the better the model’s performance.
How to Choose the Right Automatic Image Annotation Tool
With various automatic image annotation tools available, selecting the right one for your business is crucial. Here are some factors to consider:
1. Functionality
Evaluate the features offered by different tools. Look for capabilities such as object detection, image segmentation, and classification. The tool should align with your specific needs and use cases.
2. Usability
The tool should have an intuitive user interface that minimizes the learning curve for team members. A user-friendly design promotes better adoption across your organization.
3. Scalability
As your business grows, so will your data requirements. Choose a tool that can scale with your business needs effortlessly, accommodating larger datasets and additional features.
4. Integration
Your chosen tool should easily integrate with your existing software and hardware systems. This seamless integration is essential to maintain operational efficiency.
5. Customer Support
Reliable customer support can make a difference when transitioning to a new tool. Ensure the provider offers robust support, including tutorials, documentation, and responsive customer service.
Implementation of Automatic Image Annotation Tools in Software Development
After choosing the right automatic image annotation tool, it’s essential to implement it effectively into your workflow. Here’s how you can do so:
1. Define Your Objectives
Start by outlining what you hope to achieve with the tool. Whether it’s improving the speed of data preparation or enhancing the quality of your ML models, having clear objectives will guide your implementation strategy.
2. Train Your Team
Ensure that relevant team members are trained on how to use the tool. This training should encompass all functionalities, allowing team members to leverage the tool fully for better results.
3. Start with a Pilot Project
Before fully integrating the tool into all projects, consider starting with a pilot project. This will help identify any issues or areas of improvement before a larger rollout.
4. Monitor and Optimize
Once implemented, continuously monitor the performance of the tool and the impact on your workflow. Collect feedback from users and make adjustments to optimize usage.
5. Measure Outcomes
Finally, evaluate the outcomes against your original objectives. This measurement will help determine the ROI of the tool and inform future decisions regarding data annotation strategies.
Case Studies: Success Stories of Automatic Image Annotation Tools
Businesses across various industries have successfully implemented automatic image annotation tools to enhance their operations. Here are a few compelling case studies:
1. Automotive Industry
An automotive company utilized an automatic image annotation tool to enhance their self-driving car algorithms. By efficiently annotating thousands of images of roadways and obstacles, they successfully improved the accuracy of their predictive models, resulting in safer driving systems.
2. Healthcare Sector
A medical imaging company adopted the tool to assist in analyzing X-ray images. The automation of image annotation allowed radiologists to focus more on diagnostics rather than data preparation, increasing their productivity and improving patient outcomes.
3. E-commerce
An online retail giant implemented an automatic image annotation tool to enhance product image categorization. This tool enabled faster processing of product images, improving user experience and driving increased sales conversion rates.
Conclusion: The Future of Image Annotation in Business
The integration of an automatic image annotation tool represents a significant leap forward in the efficiency of software development processes. As businesses continue to harness the power of AI and machine learning, the demand for effective image annotation will only grow. By investing in cutting-edge annotation tools, companies position themselves at the forefront of innovation, ready to capitalize on opportunities in an evolving digital landscape.
In summary, the benefits of adopting an automatic image annotation tool are clear: time savings, cost efficiency, increased accuracy, and robust data generation. As you consider these tools for your own software development needs, remember that making the right choice will set the stage for your success in leveraging AI technologies effectively.